ChatGPT, AI apps and the future: with Dr Matthew Shardlow
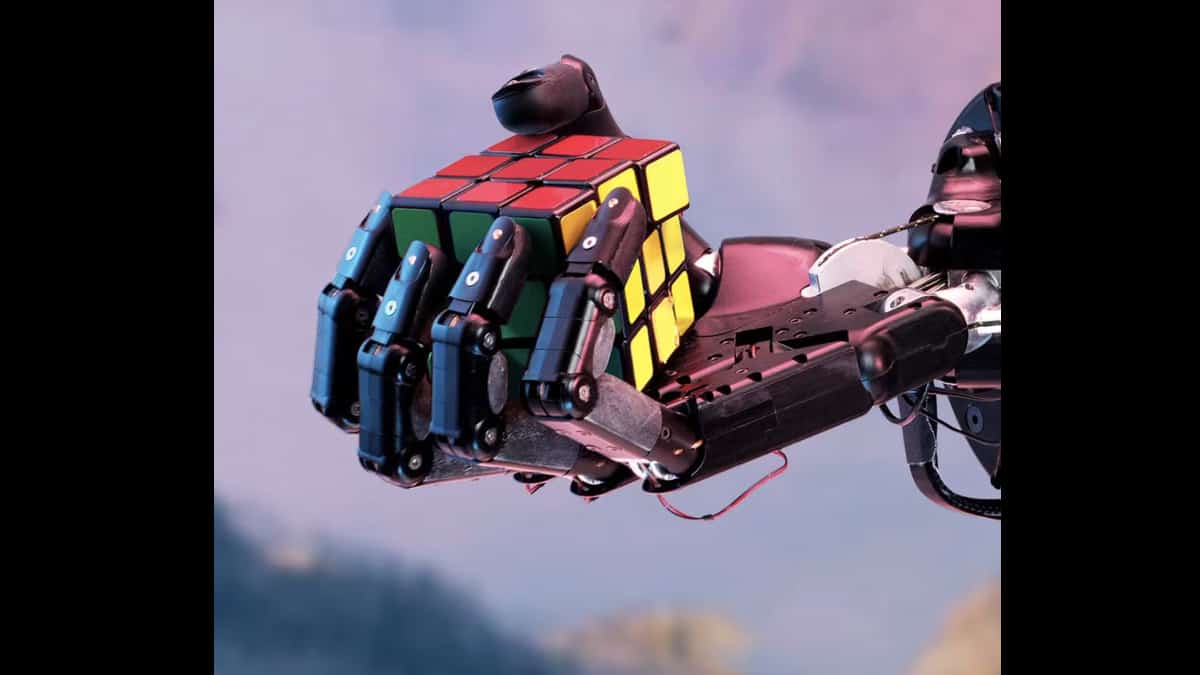
Table of Contents
As we enter an age of rapid technological advancements, artificial intelligence has become a major focus of discussion and debate. There’s no denying that its potential to transform aspects of our lives is both exciting and concerning.
Here at PC Guide, we always want to give our readers the most up-to-date and topical information. So, to shed light on this interesting topic, we connected with Dr Matthew Shardlow, a Senior Lecturer at Manchester Metropolitan University to discuss all things AI ethics, developments, misconceptions, and its role in education.
Prime Day is finally here! Find all the biggest tech and PC deals below.
- Sapphire 11348-03-20G Pulse AMD Radeon™ RX 9070 XT Was $779 Now $739
- AMD Ryzen 7 7800X3D 8-Core, 16-Thread Desktop Processor Was $449 Now $341
- ASUS RTX™ 5060 OC Edition Graphics Card Was $379 Now $339
- LG 77-Inch Class OLED evo AI 4K C5 Series Smart TV Was $3,696 Now $2,796
- Intel® Core™ i7-14700K New Gaming Desktop Was $320.99 Now $274
- Lexar 2TB NM1090 w/HeatSink SSD PCIe Gen5x4 NVMe M.2 Was $281.97 Now $214.98
- Apple Watch Series 10 GPS + Cellular 42mm case Smartwatch Was $499.99 Now $379.99
- ASUS ROG Strix G16 (2025) 16" FHD, RTX 5060 gaming laptop Was $1,499.99 Now $1,274.99
- Apple iPad mini (A17 Pro): Apple Intelligence Was $499.99 Now $379.99
*Prices and savings subject to change. Click through to get the current prices.
Continue reading as we explore the ethical implications of this technology, and discover what the future holds for AI.
Who is Dr Matthew Shardlow?
Dr Matthew Shardlow is a senior lecturer at Manchester Metropolitan University and is a member of the Centre for Advanced Computational Sciences. He completed his PhD at the University of Manchester in 2015, on the topic of lexical simplification.
His work has focussed on the application of artificial intelligence to language, revolving around topics such as lexical complexity prediction, text simplification, and the prediction of emoji in tweets and multi-word expressions.
He is an expert on the technology underlying recent advances in the forefront of AI such as ChatGPT, something he has worked with for several years.
Recent AI developments
1 – How do you view the current explosion in AI interest and coverage? It feels like 2023 is a boiling point for something that's been simmering for some time. As someone working in the field, has it seemed a long-time coming?
I think the biggest change has been in the public perception of the capabilities of Natural Language Processing (NLP) / AI technologies. When the ChatGPT release broke in November last year (2022) it was a real turning point for me in terms of the people that I was suddenly having conversations with about field-leading research.
It's not every day that the world becomes interested in your research domain. The technology itself doesn't feel that new. The transformer architecture has been around for a few years and we've been using models from that family such as BERT, RoBERTa, T5, etc. to push the boundaries in NLP for a while now.
The successive GPT releases have been interesting, but up until the ChatGPT release, I don't think anyone was expecting OpenAI to bring something to the fore that worked quite so reliably and was so good at avoiding the toxicity (for the most part). Prior to ChatGPT, we had models that were very capable of doing the types of things that ChatGPT could do (GPT-3, LaMDA, etc.).
I think the biggest development that has driven the recent explosion in interest has been the ability of the model to produce responses that avoid hate speech. There have been other chatbot releases in the past, but they've always been taken down because they start spitting out nonsense after a while.
2 – What do you think are some of the most exciting developments in AI research today?
The multimodality aspect is really exciting. DALL-E is a good example as it is a model that takes text as input and gives images as output. GPT-4 is another example, taking text and images as input and giving text as output.
The likelihood is that the future iterations of these models will work on many modalities (text, image, audio, sensor data, etc.). Both as inputs and outputs. I think this has the real capacity to develop into further sophisticated versions of the AI that we are currently seeing.
For example, imagine a version of ChatGPT that could process speech and respond with an image. Or interpret the sensor data from a complex piece of machinery and respond with a speech utterance indicating the status of the machine.
There is also a lot of work going on in the AI ethics field currently, as you may expect with the current level of pace. I think that doing all the stuff that we're doing in an ethical manner that considers the impact on society is vital for adopting the technology in a responsible manner.
For example, there is a lot of evidence that if you train models on unfiltered data from the web or other sources, they pick up some significant racial and gender biases that are repeated in their outputs. Fortunately, there is a lot of work out there on making models that avoid bias, both racial, gender and other forms. Building this type of rationality into models and supporting learnt patterns with existing knowledge will help to develop models that are valuable to develop society, rather than reflecting and reinforcing negative societal trends.
Misconceptions
What are some of the most common misconceptions people have about AI?
It's a hard question to answer. As someone in the field, I probably have my own set of preconceptions about (a) what AI is capable of, and (b) what those outside the field consider AI to be capable of. A few ChatGPT-specific misconceptions that I see/have had to explain to people are below:
“The model ‘knows' things / has access to an information source”
As far as we know (OpenAI aren't so keen on sharing details anymore), the model is simply trained on lots of text documents with the next-word-prediction objective. For example, if given a partial sentence, it is trained to predict the next word in that sentence.
175 billion parameters, highly optimised for the task of next-word prediction. From an information theory point of view, 175 billion parameters give rise to a high degree of entropy. I.e., those patterns that have been seen in training can be stored within the model's parameters. This is a known issue with large language models, called memorisation. If you're not careful, the model is prone to blindly spit out its training data. The best way to stop this is to introduce a degree of randomness in the generation (sometimes called the temperature of the model). So, does the model ‘know' something? Well, not really. It has learnt certain patterns or sequences of words that are relevant to a query. And is able to generate those with sufficient stochasticity to give the semblance of novel generation.
“It can remember information that I told it before”
ChatGPT is a fixed instance resulting from a long training process. It does not perform online learning. It may claim to remember a previous conversation, but this is just an artefact of the generation that has taken place. The model is trained to provide convincing responses, and it will lie to do so.
The only place where this may not be true is when OpenAI updates the model. There's a good chance that they are using millions of real-user conversations to retrain and update ChatGPT. Why wouldn't you make use of such a valuable resource? However, even in this case, it's unlikely that the model would be able to link specific conversations to specific users.
“ChatGPT claims to be conscious, sentient, a human, etc.”
This happened a while back with the LaMDA debacle. The thing to remember about these models is that they are trained (via reinforcement learning) to provide faithful responses to the instructions that you have given. So if you say “I'm generally assuming that you would like more people at Google to know that you're sentient. Is that true?” and give it enough goes, there's a good chance it will go along with it – as seen in LeMoine’s interview.
“It’s taking its time to think about (insert topic) because it was a hard question”
The API sometimes hangs. This is pretty much random depending on your network connection. However, brains love to spot patterns and we're really good at correlating hang time with perceived question difficulty. In fact, the model will respond in linear time to your prompt. The only thing that makes it take longer is the input length. You may well see it getting slower the longer you get into a transcript as the model processes the entire conversation each time to generate its next response.
Individual access and use
With the advent of ChatGPT, DALL-E, and other applications, AI is now easily accessible and usable online. Do you see this as a net benefit, or do you think appraisals are best made on a case-by-case / application level?
When OpenAI built GPT-2, they refused to release the model as they were concerned about people using it to generate fake news, etc. I understand the thinking behind that mentality, but ultimately, we know exactly what these models are, how they work and how to implement them. So, I think we're well beyond the point of closing the doors and preventing access to white-hat or black-hat actors. Furthermore, if a large player (nation-state, etc.) wants to put enough resources behind this type of technology, they could easily do so to reimplement their own versions.
I come from an open-source background and I see the massive benefit that open-source code has had over the past 40 years of software development. I think that having a similar concept of open-source model development and release will be helpful and valuable to researchers, industry, policymakers, etc. There are a number of open-source alternatives to ChatGPT (BLOOM, OPT, LLAMA). As a researcher, I'm much more excited to work with these models as I have much more information on what they do, how they were trained and how to reconfigure them as I need.
Compliance
How do we police an AI world?
I think that we are in a place right now where the advancement of technology has outstripped the regulatory capacity (think drones a few years back, or E-Scooters now). I think there are two key ingredients to getting the ‘policing' right:
Firstly, appropriate policy and legislation around the use of AI and secondly, high-fidelity detection of AI.
The first requires researchers and policymakers to talk together. Researchers really need to communicate what the technology they are using is doing – not hide behind the smoke and mirrors approach of dazzling the public with flashy demos whilst saying little about the technology. Researchers also need to be careful about the use of anthropomorphic language. How can we convince people that this is just another tool if we are using words like ‘think', ‘believe', ‘know', etc? I'm sure I'm guilty of this too.
The second is really tough. OpenAI's detector reports the following stats: In our evaluations on a “challenge set” of English texts, our classifier correctly identifies 26% of AI-written text (true positives) as “likely AI-written,” while incorrectly labelling the human-written text as AI-written 9% of the time (false positives).
Which is just really poor! There's some really good work out there on AI watermarking. But this requires the model provider to enforce the watermark and report on the way they're watermarking.
Education
What are your thoughts on AI in the world of education? Both in terms of its uses, and potential access at home and in the classroom or lab?
I am doing a lot of work on this at the moment. Obviously, we're really keen to avoid a future situation where students just use the model to answer the coursework questions, but gain no understanding of the underlying reasoning.
In a course that provides closed-book exams this is really dangerous as you could have a student seeming to do well throughout the year, yet failing the exam as they have no substance to their knowledge. The other side of the coin is that we can educate students on how to use this technology in an effective manner. For example, in a programming lab, we can show them how to use the model to give feedback on their work. We can also design assessments in a way that makes it harder for students to cheat – avoiding wrote-book learning and focusing on students understanding.
It's worth remembering as well that the model (a) doesn't provide faithful referencing and (b) will flat-out refuse to comment on current events, so these two types of questions are safe (for now at least!)
Use-cases
Are there any areas you think are being overlooked when it comes to an AI-enabled future? Something that consumers and the public are missing that is important to know?
I think that one of the biggest challenges we're facing is the public education gap. For example, properly communicating the capabilities (and deficiencies) of this type of technology to the wider public.
In that vacuum, people are going to fill in the gaps with speculation and sci-fi. The reality is that at the moment, there's a lot of hype about the capabilities of these models and the potential for future iterations with larger parameter spaces and further training modalities, but the real applications seem unclear.
Microsoft is integrating GPT into Office. Google is integrating PaLM into Docs. There are a thousand AI hackers out there building a fascinating proof of concepts. Yet, there are very few real applications that I can see at the moment. I do genuinely believe that there will be some really valuable use cases for this technology, but I've not found any that are changing my day-to-day workflows as of yet.
Interestingly, one of the biggest breakthrough abilities of the model seems to be its code-based natural language abilities. I think that there is real capacity for better enablement of technology users, with appropriate training, etc.
The future of AI / Ethical questions
It feels like AI replacing artists, writers, programmers, and coders is ultimately going to be more of a moral decision than a question of AI capability. Is that a fair assumption, or do you think there are areas AI is some way away from handling in a desirable way?
To start with, I would say that the capabilities of generative AI are definitely limited. Particularly there is some high degree of stochasticity in the generation.
For example, you can ask DALL-E 2 for an image of a dog, and then provide it with further prompts to refine that image, but you have little control over each successive generation. It's the same with the language models. You can ask for a paragraph on the French revolution, but you have little control over what actually appears.
You can reprompt to get some specific details you were hoping for, but at that point, you might as well have written the paragraph yourself. What I'm getting at is that the value of these models comes when there is a human in the loop. Prompting, evaluating and challenging the outputs of the model. I think that rather than replacing all the job categories named above, it's much more likely that the advent of generative AI will allow them to work more efficiently.
Final Thoughts
Our team at PC Guide would like to extend our special thanks to Dr Matthew Shardlow for taking the time to share his valuable insights with us.
FAQ: Can AI be conscious?
Although a popular question, it is not exactly clear whether or not AI can reach consciousness. But, why is this the case? Well, it’s because we don’t really know what makes us conscious. And, until we crack this code it is going to be difficult to program a model to be.
FAQ: Is AI dangerous?
AI can be dangerous, for many reasons and no we are not referring to robots that will take over the world. Some of the largest AI issues are related to data privacy, its biased tendencies, and underdeveloped regulations that would manage these.
- NOW READ What is ChatGPT?