Best CPUs for deep learning in 2025 – our top picks for AI
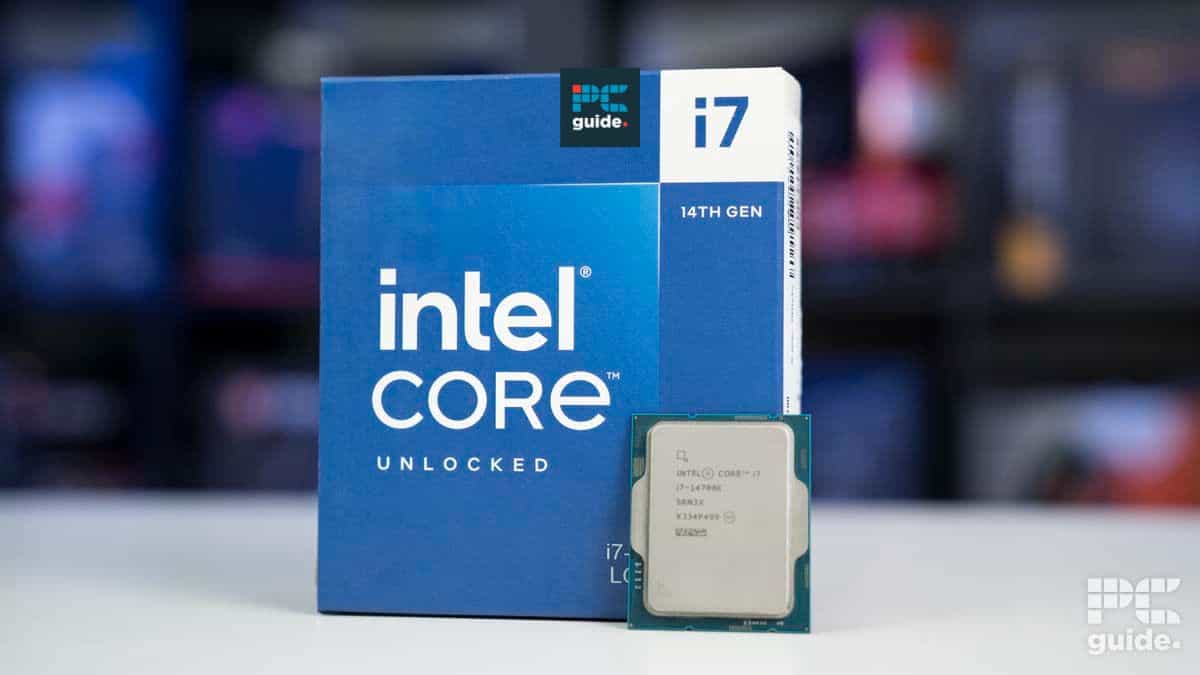
Table of Contents
Choosing the right CPU (central processing unit) for your deep learning project can make all the difference in terms of efficiency, speed, and accuracy. In this article, we’ll explore the best CPUs for you and then discuss some of the key features to look for when making your decision.
The ever-expanding field of deep learning has been transforming various industries, including healthcare, finance, and autonomous vehicles. As a result, there is an increasing demand for high-performance computing to handle the intensive computational workloads necessary for training and inference in deep learning systems. At the heart of any deep learning system is a powerful CPU that can handle the intense computational workloads required for training and inference.
Prime Day is finally here! Find all the biggest tech and PC deals below.
- Sapphire 11348-03-20G Pulse AMD Radeon™ RX 9070 XT Was $779 Now $739
- AMD Ryzen 7 7800X3D 8-Core, 16-Thread Desktop Processor Was $449 Now $341
- ASUS RTX™ 5060 OC Edition Graphics Card Was $379 Now $339
- LG 77-Inch Class OLED evo AI 4K C5 Series Smart TV Was $3,696 Now $2,796
- Intel® Core™ i7-14700K New Gaming Desktop Was $320.99 Now $274
- Lexar 2TB NM1090 w/HeatSink SSD PCIe Gen5x4 NVMe M.2 Was $281.97 Now $214.98
- Apple Watch Series 10 GPS + Cellular 42mm case Smartwatch Was $499.99 Now $379.99
- ASUS ROG Strix G16 (2025) 16" FHD, RTX 5060 gaming laptop Was $1,499.99 Now $1,274.99
- Apple iPad mini (A17 Pro): Apple Intelligence Was $499.99 Now $379.99
*Prices and savings subject to change. Click through to get the current prices.
Whether you're just starting out or are a seasoned data scientist, this guide will help you find the perfect processor for your next AI/DL project. The fact of the matter is that across deep learning for large-scale storage, data science, and data analytics, you’ll need a reliable CPU that can take a bit of heat. We’ve lined up four solid options from both Team Red and Team Blue, so no matter your preference, you should have your needs covered.
Products at a glance
-
Best CPU for deep learning
Intel Core i9-13900K
-
Best AMD CPU for deep learning
AMD Ryzen 9 7950X3D
- Cores: 16
- Thread: 32
- Base Clock Speed: 100 MHz
- Boost Clock Speed: 5.7 GHz
- L3 Cache: 128 MB (shared)
- TDP: 120 W
-
Best CPU for deep learning for the money
Intel Core i7-14700K
- Cores: 20 (8P-12E)
- Threads: 28
- Boost clock speed: P-Core 5.5GHz / E-Core 4.3GHz
- Base clock speed: P-Core 2.5GHz / E-Core 3.4GHz
- L3 Cache: 33 MB
- TDP: 125W
-
AMD Ryzen 9 7900X
- Cores: 12
- Threads : 24
- Boost clock speed: 5.6 GHz
- Base clock speed: 4.7 GHz
- L3 Cache: 64 MB
- TDP: 170 W
How did we pick the best CPU for deep learning?
When it comes to selecting the best CPU, there are several key factors to consider. First and foremost, the CPU's core count and clock speed are critical in ensuring the fast and efficient processing of data.
Deep learning involves working with massive datasets and having a high core count and clock speed can significantly reduce the time it takes to train models. Additionally, a large cache and high memory bandwidth are essential for minimizing data retrieval time and enabling fast data transfer between the CPU and memory.
Another critical consideration when selecting a CPU is its compatibility with popular deep-learning libraries such as TensorFlow, PyTorch, and Keras. These libraries are widely used in the data science community, and having a CPU that can effectively support them can streamline the development process.
What’s more, the CPU's ability to support the complexity of vectorization is crucial in ensuring the efficient processing of large datasets. Low-latency interconnects are also vital for enabling efficient communication with other hardware components in the system, such as GPUs or RAM.
While weighing in on the specs and heavy details, matters such as budget and affordability never left our minds either. There’s a whole world to dive into with professional CPUs such as the AMD Threadripper Pro series, but this time around, we just wanted to provide consumer-focused picks.
We also made sure to lean heavily on our hands-on experience and cherry-picked the perfect lineup that we’ve approved in our in-house reviews from the PC Guide testing lab.
If you want to learn more about deep learning, then head over to our Machine Learning vs Deep Learning in AI guide. Of course, in AI/DL scenarios, the graphics card still does a lot of the heavy lifting overall, so it’s definitely worth running your eyes over our picks of the best GPU, too.
- Cores: 24 (8P-16E)
- Threads: 32
- Boost speed : P-Core 5.8GHz / E-Core 4.3GHz
- Base speed: P-Core 3.0GHz / E-Core 2.2GHz
- L3 Cache: 36 MB
- TDP: 253 W
- Platform: Intel Socket 1700
- Boost speeds of up to 6 GHz, and overclockable too
- Leading multi-threading performance
- Premium binned silicon
- Still premium price, despite being replaced by the 14900K
- Performance comes with high power consumption, of 253W
The Intel Core i9-13900K is widely regarded as one of the best CPUs for deep learning. Its processing power is so impressive that it can even rival AMD Threadripper CPUs, making it unnecessary to opt for one of the Pro-level products.
One of the most significant advantages of the 13900K is its 20 PCIe express lanes, which can increase even further with a Z690/Z790 motherboard. This is crucial since many deep learning tasks rely on the GPU, and these extra lanes provide more power for GPU acceleration.
Additionally, this processor boasts an impressive hybrid architecture: 24 cores, 32 threads, and 36MB of L3 cache. With the ability to boost up to 6GHz, this CPU delivers unparalleled performance for a consumer-grade processor.
While the Intel Core i9-13900K is no longer the flagship it was at release, generous discounts and high performance still make it an ideal processor to consider in 2025.
PC Guide
It’s important to note though, that this is a relatively expensive chip, and it also requires adequate cooling due to its power consumption of 253W. However, neither of these factors was enough to deter us from awarding it a commendable 4 stars in our i9-13900K review.
Despite these factors, for serious professionals, the Intel Core i9-13900K is undoubtedly a worthwhile investment. Its exceptional processing power, compatibility with deep learning libraries, and additional PCIe express lanes make it an ideal choice for those tasks.
What users say
On Amazon, the Intel Core i9-13900K has a rating of 4.6 stars, and more than 1,400 people have rated the product. As per the reviews, customers seem pleased with the CPU’s performance. They mention that the processor is fast, renders projects fast, and has lots of fast PCIe lanes. However, users had mixed opinions on thermal management.
- Cores: 16
- Thread: 32
- Base Clock Speed: 100 MHz
- Boost Clock Speed: 5.7 GHz
- L3 Cache: 128 MB (shared)
- TDP: 120 W
- Platform: AMD Socket AM5
- Compatible with PCI Express 5.0 for high-speed data transfer
- Higher boost frequencies, and loads of L3 cache
- Overclockable for even faster speeds
- Requires a compatible AM5 socket
- Higher TDP (170W) may require more power and cooling
- Definitely on the pricey side, more worth it when at discount
The AMD Ryzen 9 7950X3D is a powerful flagship CPU from AMD that is well-suited for deep learning tasks, and we raved about it highly in our Ryzen 9 7950X3D review, giving it a generous 4.5 stars.
With its Zen 4 architecture and TSMC 5nm lithography, this processor delivers exceptional performance and efficiency. It boasts 16 cores and 32 threads, allowing for efficient parallel processing and enabling faster data processing for deep learning algorithms.
The AMD Ryzen 9 7950X3D is far from the cheapest chipset on the market, but with unparalleled performance, it's also one of the best.
PC Guide
The Ryzen 9 7950X3D's base frequency of 4.5 GHz and boost frequency of 5.7 GHz provide outstanding clock speeds for speedy computations, while the massive 64MB L3 cache provides ample storage for data manipulation. This processor's TDP of 170W is relatively high, but this is to be expected from a high-performance processor that can handle demanding workloads.
Moreover, the Ryzen 9 7950X3D is compatible with the latest Socket AM5, ensuring that it will work with the latest motherboards. The launch MSRP of $699 is a reasonable price point for a high-end CPU that delivers the level of performance required.
What users say
The AMD Ryzen 9 7950X3D has a 4.8-star rating, and more than 650 people have rated the processor. Most of the positive reviews mentioned that they were pleased with the CPU’s performance, speed, and build quality. They mentioned that the CPU worked well, ran cool, and was silent. However, customers have mixed opinions on the price of the product.
- Hybrid technology performs great
- Leading for creativity, productivity, and data analysis
- Its pricing is identical to previous generation
- As a ‘refresh’, there’s not much of a leap from 13700K
- Thread count could be higher considering the price
- Technically, there are more affordable options available
For those who are a bit tighter for money or concerned about power consumption, the Intel Core i7-14700K is an excellent option for deep learning. Unlike other Intel CPUs, this chip delivers excellent performance and even outperforms Intel's own 12th-Gen Core i9-12900K – the previous flagship.
While there are cheaper options available, we don’t particularly recommend compromising on processing power by going for something like the Ryzen 5 7600X or the Core i5 13600K.
The 14700K is a great middle-ground for those looking to game and work on the same PC. This CPU has a plethora of cores and threads at its disposal to chew through basically any workload you can throw at it.
PC Guide
These processors may save you money, but you'll miss out on higher clock speeds and find yourself lacking in overall processing power. It’s priced competitively for its performance offering, but it’s fairly widely agreed that the 14th gen doesn’t improve a lot over the 13th gen overall.
The Core i7-4700K is a great value-for-money option that offers high clock speeds of up to 5.6 GHz with Turbo Boost Max Technology 3.0 and is also easier to cool than the more powerful i9-13900K. It delivers excellent performance for both gaming and productivity and provides an affordable alternative to more expensive CPUs; at least, that was our general takeaway from our hands-on i7-14700K review.
What users say
The Intel Core i7-14700K has been rated by more than 1,000 people, and it has a rating of 4.6-star on Amazon. Most customers were happy with the performance and price of the CPU. “So far so good. This CPU is a monster and runs very nicely and boosts to max clocks,” one of the customers wrote in a review. Although most reviews were positive, a few customers said that they had heating issues.
- Cores: 12
- Threads : 24
- Boost clock speed: 5.6 GHz
- Base clock speed: 4.7 GHz
- L3 Cache: 64 MB
- TDP: 170 W
- Platform: AMD Socket AM5
- The strongest single- and multi-thread performance
- High boosted frequencies, and overclockable too
- DDR5 and PCIe 5.0, and very future proof as it's the first generation on AM5
- 7950X provides better multi-core performance for not much more money
- Does not come with a stock cooler, and runs on the hot side
- Needs an AM5 motherboard, costs can add up
The Ryzen 9 7900X is not only a great option for gaming and desktop applications but is also one of the best deep-learning CPUs. Its 12 cores and high clock speeds make it a great choice for handling large datasets and intense computational workloads.
The Zen 4 architecture provides significant improvements in instruction per clock (IPC), which translates into faster processing times and more accurate results.
Furthermore, the Ryzen 9 7900X has been designed with deep learning in mind. It features a high-end core architecture that provides great performance for neural networks and other machine-learning tasks.
This CPU can work hard, and play hard too. It is just as comfortable gaming as it is in workstation and productivity scenarios, a real jack of all trades, if you will.
PC Guide
This makes it a versatile option that can handle a wide range of applications beyond gaming and desktop productivity, and these were features that we praised in our Ryzen 9 7900X review where we described it as a “jack of all trades”.
In conclusion, the Ryzen 9 7900X is a powerful and affordable CPU that offers excellent performance for deep learning tasks. With its combination of high clock speeds, multi-core architecture, and Zen 4 technology, it is a great option for those looking to build a high-performance system without breaking the bank.
What users say
On Amazon, the AMD Ryzen 9 7900X has a rating of 4.7 stars, and over 1,270 people have rated this product. As per the customer reviews, the CPU seems to have impressed the users with its performance and easy installation. They mentioned that it runs games well. However, they had mixed opinions on temperature.
Features and considerations
The first thing to consider is how demanding a PC you'll be looking to build. For demanding professional workloads, it is advisable to opt for AMD Threadripper or Intel Xeon W CPUs. These CPUs are preferred because they offer more lanes to accommodate multiple GPUs, making them the ideal choice for serious professional workloads.
If those are not the cases and you don't need a CPU with multiple GPU lanes, then you'll need to choose a strong GPU with a high core count to enable parallel processing of large amounts of data. The high CPU cores are the more crucial aspect to consider. Once that's taken care of, you'll need a high-frequency CPU so the unit can perform multiple calculations fast.
So far, these are the usual requirements for a high-end CPU. To be a bit more specific about what you'll be looking for, consider low-latency interconnects. The CPU should enable efficient communication with other system components, such as graphics cards or memory.
Moreover, the CPU should support popular deep learning libraries such as TensorFlow, PyTorch, and Keras that have a focus on artificial and deep neural networks.
To complete your PC setup, consider investing in a GPU with NVIDIA tensor cores. Tensor cores are specifically designed to perform matrix operations, which are a key part of deep learning algorithms.
If you are looking for more recommendations, then head over to our best laptops for programming articles.
Do I need a good CPU for deep learning?
Yes, you definitely need a good CPU for deep learning. These AI models are computationally intensive and require a lot of processing power to train and run. Ensuring you invest in a high-quality CPU will help speed up the training process and improve your models’ performance.
Should I get an AMD or Intel CPU for deep learning?
Obviously, user preference has a huge role in answering this question, and it will differ from person to person. However, while Intel chips can beast-mode some deep learning tasks and have a wide variety of RAM, often getting this performance means that they will guzzle through your power and be quite demanding.
On the other hand, AMD processors might be a bit more particular with their RAM usage, but you might find they have the capability to perform really highly with very little power consumption.