A generative adversarial network (GAN) is a machine learning framework with two neural networks. These compete against each other through deep learning methods in order to produce more accurate predictions.
The two neural networks of a GAN machine AI are; the discriminator and the generator. The discriminator is a deconvolutional neural network, while the generator is a convolutional neural network.
The generator’s main objective is to create outputs that could be mistaken for real data in an artificial way. On the other hand, the discriminator’s goal is to identify the outputs received which have been artificially created by the generator.
Essential AI Tools
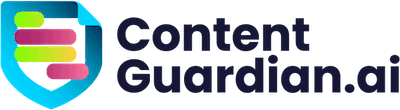
Content Guardian – AI Content Checker – One-click, Eight Checks
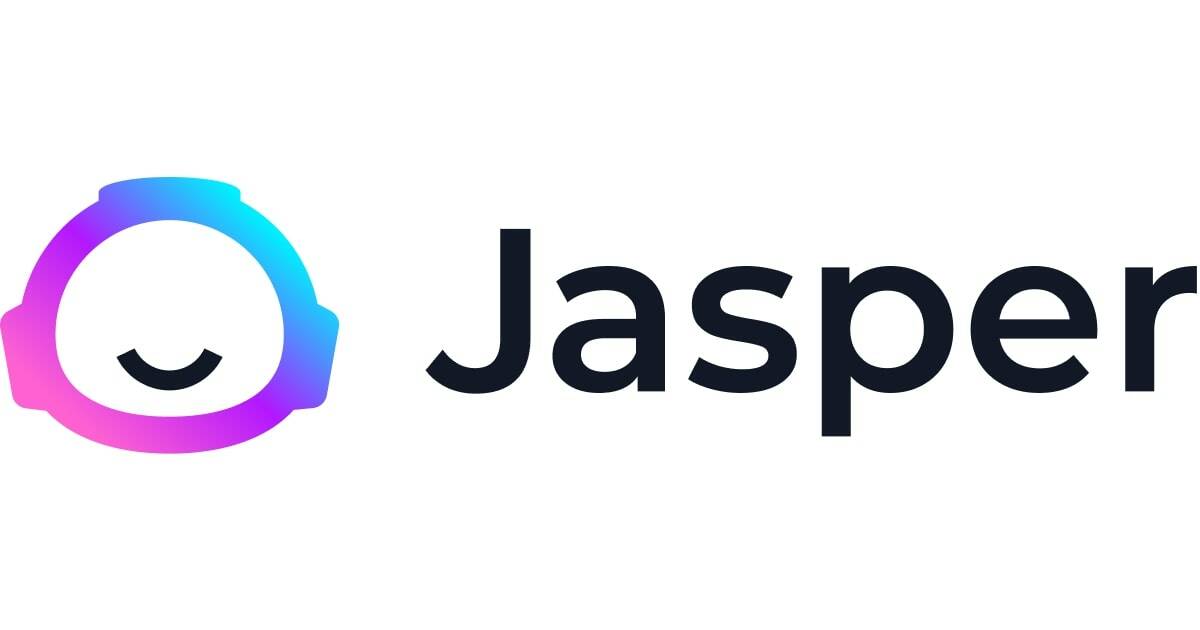
Jasper AI
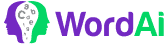
WordAI
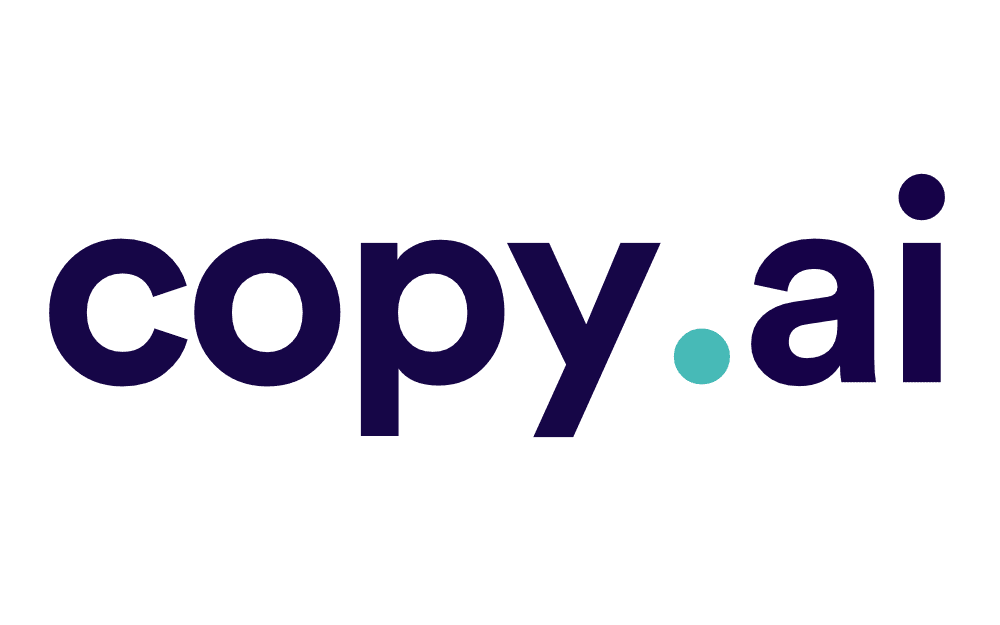
Copy.ai
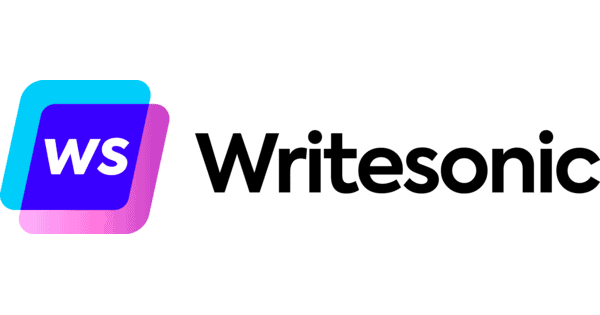
Writesonic
How do GANs work?
The generator is trained to create fake data, while the discriminator is built to identify the fake data created by the generator as well as true examples. Whenever the discriminator identifies the fake data produced by the generator, the generator suffers a penalty.
Generative adversarial networks are divided into 3 different categories – generative, adversarial, and networks. Now, let’s look at each of them one after the other.
Generative – Describes the process by which data is created using a problematic model.
Adversarial – This refers to models trained in adversarial settings.
Networks – Deep neural networks may be used as AI algorithms for the purpose of training.
Types of Generative Adversarial Networks (GANs)
GANs come in different types and serve different purposes. Some of the most common GAN types are listed below.
Conditional GAN
Using class labels, this kind of GAN allows the conditioning of the network with specific and new information.
Vanilla GAN
Vanilla GAN is the simplest kind of GAN. By using stochastic gradient descent, its algorithms optimize the mathematical equation. Vanilla GAN consists of a discriminator as well as a generator. The creation and classification of images created is done using the discriminators and generators as direct multi-layer perceptrons.
Super Resolution GAN
This type of GAN allows a low-resolution image to be transformed into a well-detailed one. It fills in blurry spots to increase the image resolution.
Deep Convolutional GAN
This type of GAN produces high-resolution images by using a deep convolutional neural network.