JARVIS-1 is an artificial intelligence project pushing the boundaries of LLM’s. Devised by research students from various Universities, this open-world multi-task agent gives us all an insight into free will, through the world’s favorite videogame Minecraft. Able to choose its own path, and perform its own tasks, how would a multimodal language model interact with a world of complex rules and systems not too dissimilar from our own?
What is Jarvis-1?
JARVIS-1 is an open-ended multi-task agent, essentially the closest thing to free will inside your computer. Left to it’s own devices, it will decide which tasks are necessary to complete a set goal, and will execute those tasks within the confines of a set of rules. In this case, those rules are that of Minecraft.
It uses memory-augmented multimodal language models, an extremely advanced yet fundamentally similar technology to OpenAI’s ChatGPT model, GPT-4. This is exciting because this, it seems, it all an AI agent needs to perform over 200 varying and complex tasks with “perfect” accuracy. One such task sees the AI agent asked to “cook chicken“, despite not being provided with any raw chicken, nor the furnace and fuel required for cooking it.
What is a multimodal language model?
To explain how we got here, technologically, we can follow OpenAI’s own progress with its proprietary language model tech. GPT-4 was largely the same but better, iterating on GPT-3 with a larger data set, more recent information, higher parameter count and so on – but ultimately still and LLM, or large language model.
Then, GPT-4V came out. This new neural network was a VLM, or Visual Language Model. Now, it could respond to visual inputs, and interpret them with computer vision. It could tell you how many apples or oranges were in a photo of a fruit basket, for example.
At roughly the same time as ChatGPT saw image input functionality (so to speak), it also received image output capability thanks to DALL-E 3 integration. This all serves to demonstrate how multimodality is naturally built up over time, evolving from an LLM to an MLM (the good kind?).
Essential AI Tools
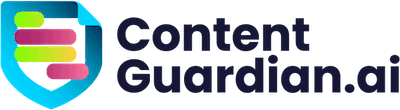
Content Guardian – AI Content Checker – One-click, Eight Checks
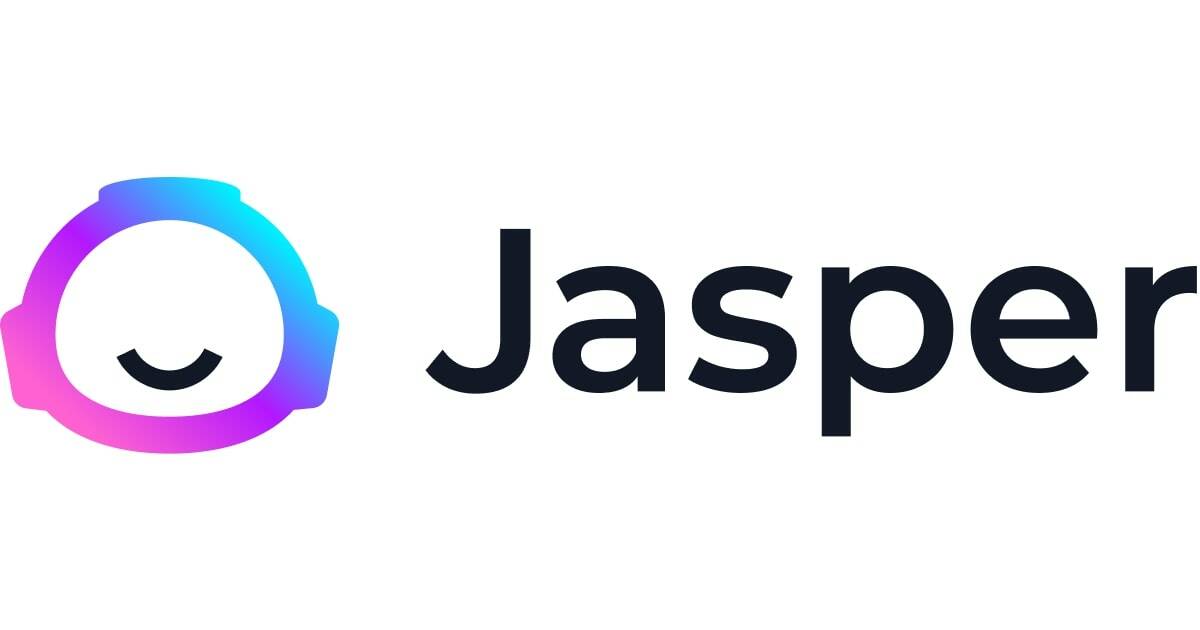
Jasper AI
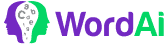
WordAI
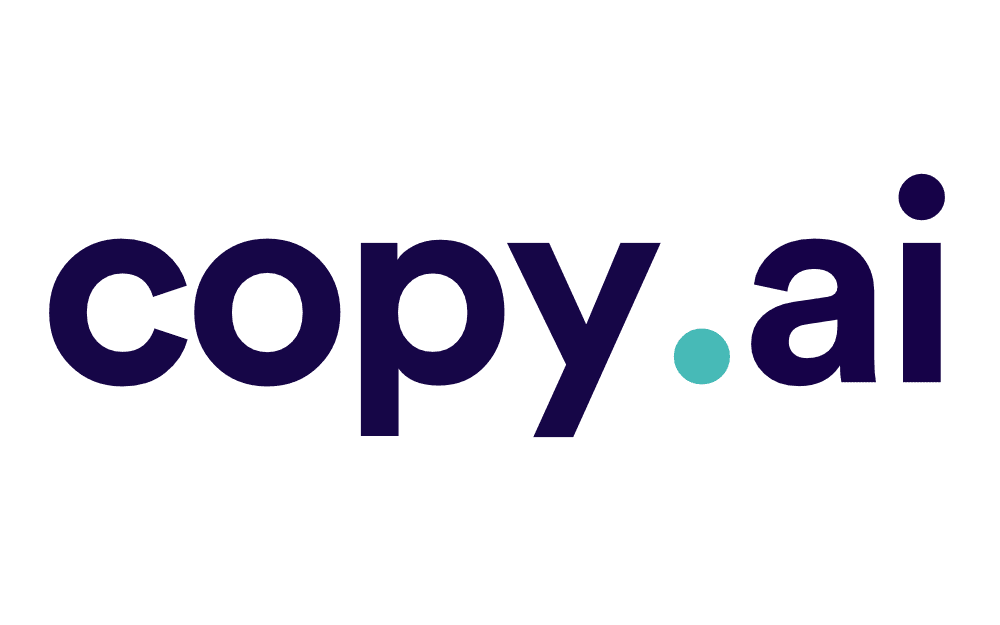
Copy.ai
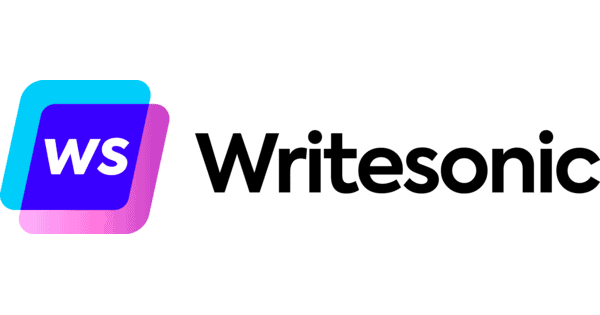
Writesonic
How Minecraft sets the stage for an open-world multi-task AI agent
In the research paper, published on November 10th by PhD candidate Zihao Wang and peers, the complexity of this AI agent is broken down somewhat. “Achieving human-like planning and control with multimodal observations in an open world is a key milestone for more functional generalist agents. Existing approaches can handle certain long-horizon tasks in an open world. However, they still struggle when the number of open-world tasks could potentially be infinite and lack the capability to progressively enhance task completion as game time progresses,” explains Wang.
The team researching this project includes Wang himself in addition to Shaofei Cai, Anji Liu, Yonggang Jin, Jinbing Hou, Bowei Zhang, Haowei Lin, Zhaofeng He, Zilong Zheng, Yaodong Yang, Xiaojian Ma, and Yitao Liang. Together, they “introduce JARVIS-1, an open-world agent that can perceive multimodal input (visual observations and human instructions), generate sophisticated plans, and perform embodied control, all within the popular yet challenging open-world Minecraft universe. Specifically, we develop JARVIS-1 on top of pre-trained multimodal language models, which map visual observations and textual instructions to plans. The plans will be ultimately dispatched to the goal-conditioned controllers.”
Circling back to the multimodality explained earlier, this AI agent utilizes “a multimodal memory, which facilitates planning using both pre-trained knowledge and its actual game survival experiences.”
In our experiments, JARVIS-1 exhibits nearly perfect performances across over 200 varying tasks from the Minecraft Universe Benchmark, ranging from entry to intermediate levels.
Zihao Wang, “JARVIS-1: Open-World Multi-task Agents with Memory-Augmented Multimodal Language Models”
Achieving a 12.5% completion rate on a particular task known as the “long-horizon diamond pickaxe task”, Jarvis 1 actually performed as much as 5x better than previous records. This achievement demonstrates the ability of artificial intelligence to learn, self-improve, and continually improve on that learning, indefinitely. Despite the unassuming nature of a video game record being broken, this represents an impressive step towards artificial general intelligence and improved autonomy in AI gents.